Fundamentals of Statistical Analysis
Statistician Zone
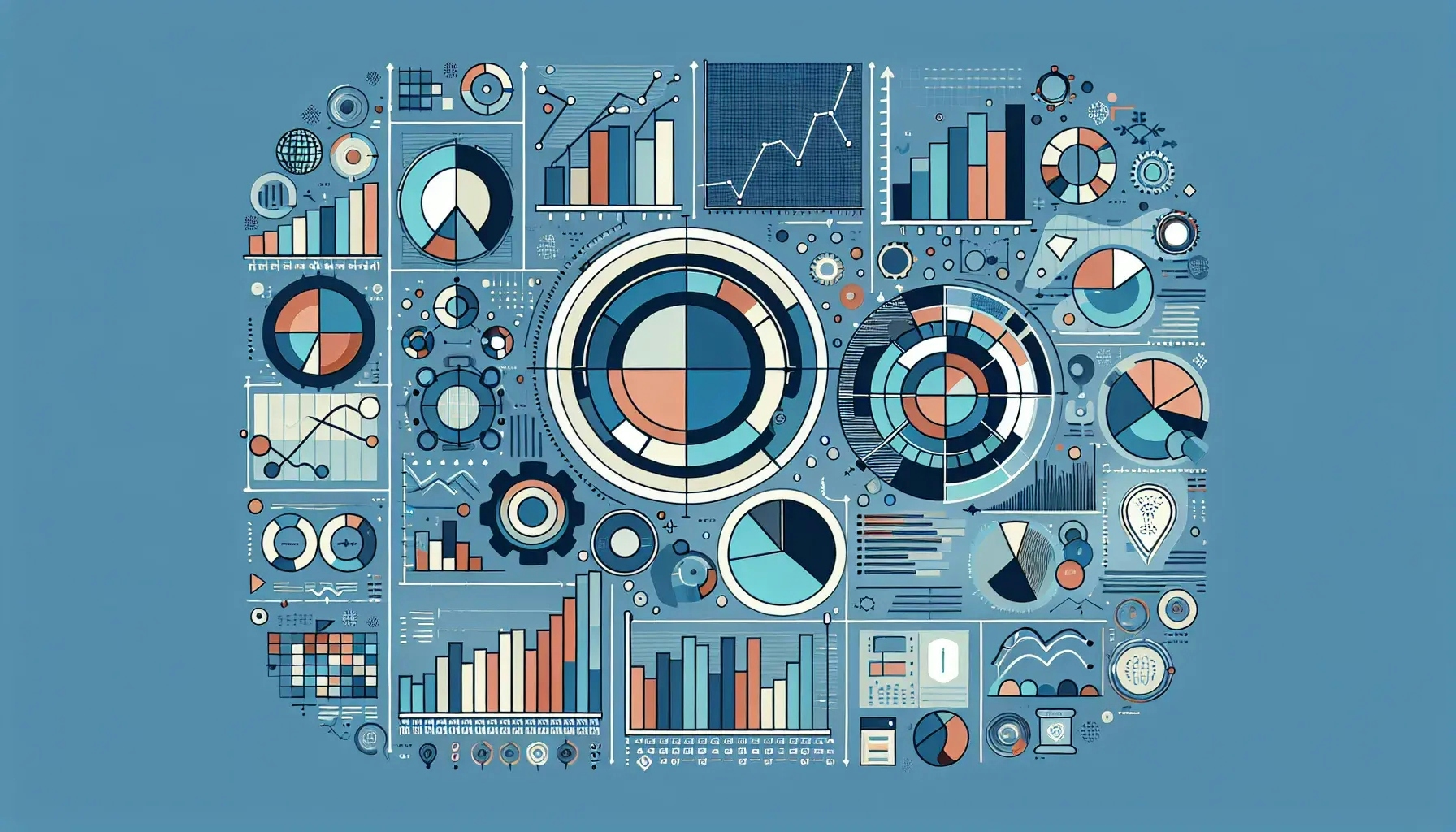
Welcome to a comprehensive exploration of the fundamentals of statistical analysis. This blog post aims to demystify the key concepts, techniques, and applications of statistical analysis. Whether you're a student, a researcher, or a professional looking to enhance your data-driven decision-making skills, this guide will provide you with the necessary knowledge and insights. Let's dive into the world of statistics and discover its transformative power.
The Essence of Statistical Analysis
Statistical analysis stands as a powerful tool that allows us to extract meaningful insights from data. It involves the collection, organization, interpretation, presentation, and modeling of data. By applying statistical analysis, we can understand trends, patterns, and relationships within data sets, which are often not apparent at first glance.
The process begins with data collection. This step involves gathering relevant data from various sources. The data can be quantitative (numerical) or qualitative (categorical). The quality of data collected significantly impacts the accuracy of the analysis. Therefore, it's crucial to ensure that the data is reliable, accurate, and unbiased.
After collecting data, the next step is data organization. This involves arranging the data in a systematic manner, making it easier to work with. Data can be organized in tables, charts, or graphs, depending on the nature of the data and the analysis to be conducted.
Interpreting data is the next crucial step. This involves analyzing the organized data to draw conclusions. Various statistical methods and techniques are used in this process, including descriptive statistics, inferential statistics, regression analysis, and hypothesis testing, among others.
The interpretation of data is followed by data presentation. This involves presenting the results of the analysis in a manner that is easy to understand. Data visualization techniques, such as graphs, charts, and infographics, are commonly used in this process.
Finally, data modeling involves creating statistical models that represent the data and the underlying relationships. These models can be used to make predictions or to understand the impact of different variables on the outcome.
Descriptive Statistics: The First Step
Descriptive statistics serve as the first step in statistical analysis. They provide a summary of the data, giving a clear and concise overview of its main characteristics. Measures of central tendency and measures of dispersion are the two main types of descriptive statistics.
Measures of central tendency include the mean, median, and mode. The mean is the average of all the data points. The median is the middle value when the data is arranged in ascending or descending order. The mode is the most frequently occurring value in the data set.
Measures of dispersion, on the other hand, provide information about the spread of the data. They include the range, variance, standard deviation, and interquartile range. The range is the difference between the highest and lowest values in the data set. Variance measures how far each data point is from the mean. Standard deviation is the square root of the variance, and it represents the average distance of each data point from the mean. The interquartile range is the range of the middle 50% of the data.
Descriptive statistics provide a solid foundation for further statistical analysis. They help us understand the basic features of the data, which is crucial for making informed decisions.
Inferential Statistics: Beyond the Data
While descriptive statistics provide a summary of the data, inferential statistics allow us to make predictions or generalizations about a population based on a sample. They provide the basis for hypothesis testing, correlation analysis, regression analysis, and more.
Hypothesis testing is a method used to make inferences about a population parameter. It involves stating a null hypothesis and an alternative hypothesis. The null hypothesis is a statement of no effect or no difference, while the alternative hypothesis is a statement of an effect or a difference. Statistical tests are then used to determine whether to reject or fail to reject the null hypothesis.
Correlation analysis is used to determine the relationship between two variables. The correlation coefficient, which ranges from -1 to 1, indicates the strength and direction of the relationship. A positive correlation indicates that the variables increase or decrease together, while a negative correlation indicates that one variable increases as the other decreases.
Regression analysis, on the other hand, is used to predict the value of one variable based on the value of another. It involves fitting a regression line to the data, which best represents the relationship between the variables.
The Power of Data Visualization
Data visualization is a key aspect of statistical analysis. It involves presenting data in a graphical or pictorial format, making it easier to understand and interpret. Data visualization allows us to see patterns, trends, and outliers that might not be apparent in raw data.
There are various types of data visualizations, including bar charts, pie charts, line graphs, scatter plots, and more. Each type of visualization is suited to a particular kind of data and analysis.
Bar charts are used to compare quantities across different categories. Pie charts show the proportion of each category in a whole. Line graphs are used to show trends over time. Scatter plots are used to show the relationship between two variables.
Data visualization is not just about creating pretty graphs. It's about telling a story with data. A well-designed visualization can communicate complex data in a simple and intuitive way, making it a powerful tool for decision-making.
Statistical Software: Tools of the Trade
In today's digital age, various statistical software tools have been developed to simplify the process of statistical analysis. These tools provide a wide range of functionalities, from basic descriptive statistics to advanced predictive modeling.
Some of the most popular statistical software tools include R, Python, SPSS, SAS, and Excel. R and Python are open-source programming languages that are widely used for statistical analysis and data science. They have a large community of users and a vast library of packages for various statistical methods.
SPSS and SAS, on the other hand, are commercial software packages that are widely used in academia and industry. They provide a user-friendly interface and a wide range of statistical procedures.
Excel, while not as powerful as the other tools, is widely used for basic data analysis and visualization. Its simplicity and familiarity make it a popular choice for many users.
The Importance of Statistical Literacy
In an increasingly data-driven world, statistical literacy has become a crucial skill. It involves understanding and interpreting statistical information, as well as making informed decisions based on this information.
Statistical literacy is not just about knowing how to perform calculations. It's about understanding the underlying concepts and principles. It's about knowing how to ask the right questions, how to collect and analyze data, and how to interpret and communicate the results.
Statistical literacy is essential in various fields, including business, healthcare, education, and government. It enables us to make sense of the vast amounts of data that we encounter daily, and to use this data to make informed decisions.
Wrapping Up: The Journey Through Statistical Analysis Fundamentals
We've embarked on a comprehensive journey through the fundamentals of statistical analysis. We've explored the key concepts, techniques, and applications, from descriptive statistics to inferential statistics, data visualization, and statistical software. We've also highlighted the importance of statistical literacy in today's data-driven world. Remember, statistical analysis is not just about crunching numbers. It's about making sense of data and using it to make informed decisions. So, keep exploring, keep learning, and let the power of statistics guide your decision-making process.