Integrating AI With Statistical Methods
Statistician Zone
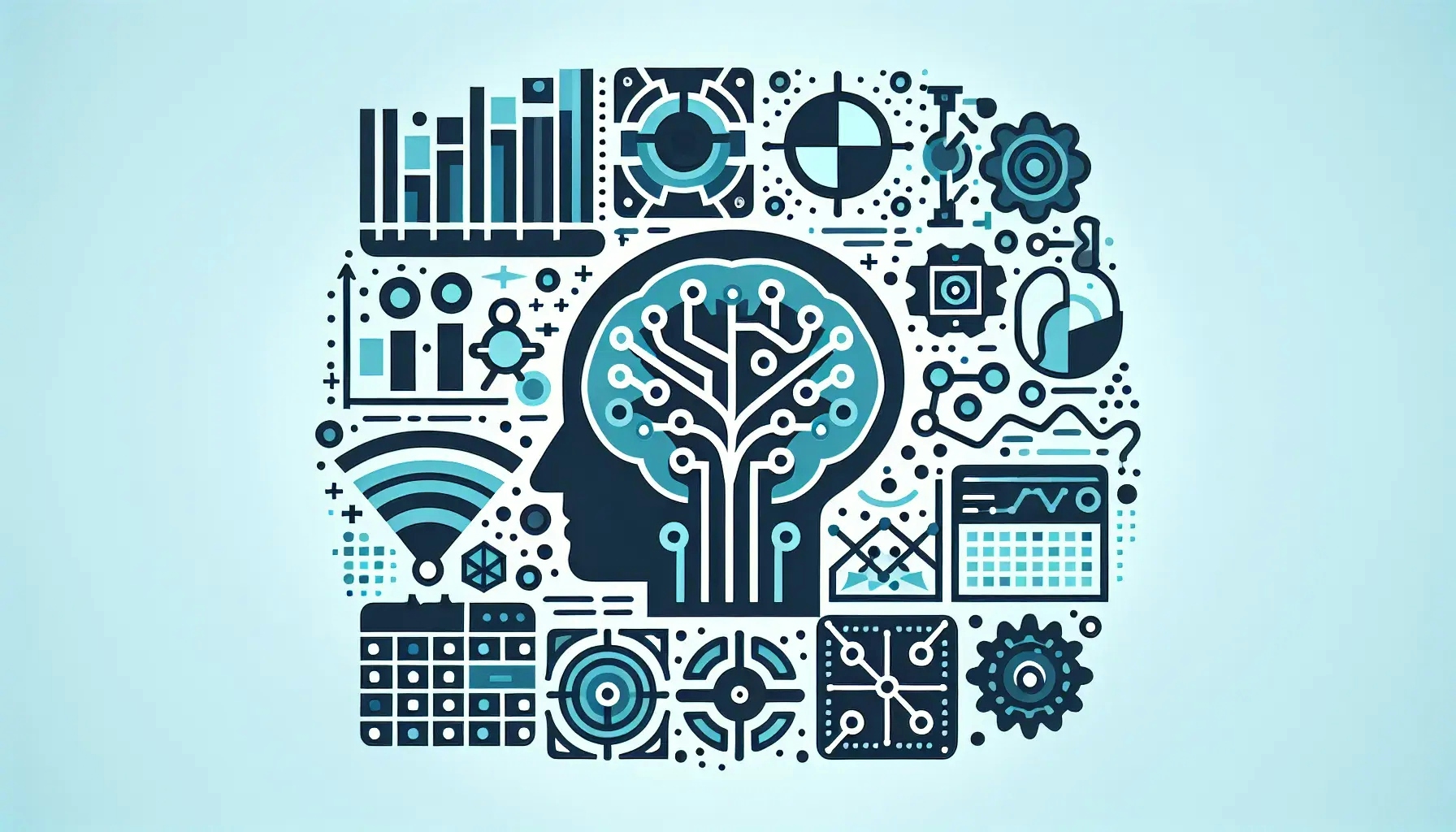
The world of data science is evolving at an unprecedented pace. One of the most exciting developments is the integration of artificial intelligence (AI) with traditional statistical methods. This fusion is creating new opportunities and challenges for data scientists and statisticians alike. This blog post will delve into the intricacies of this integration, discussing its benefits, potential pitfalls, and how to effectively implement it in your own work.
The Convergence of AI and Statistics
The integration of AI and statistical methods is not a new concept. However, recent advancements in technology and data availability have made this integration more feasible and beneficial. AI, with its ability to learn from data and make predictions, complements traditional statistical methods, which focus on understanding and interpreting data.
AI and statistical methods each have their strengths. AI excels at handling large datasets and making accurate predictions. On the other hand, statistical methods provide a solid foundation for understanding the underlying structure of data. By integrating these two approaches, we can leverage the strengths of both to gain deeper insights from our data.
However, integrating AI with statistical methods is not without its challenges. One of the biggest hurdles is the difference in the underlying philosophies of the two approaches. AI focuses on prediction and automation, while statistics emphasizes understanding and interpretation. This difference can lead to conflicts when trying to integrate the two.
Despite these challenges, the benefits of integrating AI with statistical methods are significant. This integration can lead to more accurate predictions, deeper insights, and more efficient data analysis. In the following sections, we will delve deeper into these benefits and how to achieve them.
Benefits of Integrating AI with Statistical Methods
The integration of AI with statistical methods offers several benefits. One of the most significant is the ability to handle large datasets. Traditional statistical methods can struggle with large datasets, leading to inefficient analysis and inaccurate results. AI, on the other hand, excels at handling large datasets, making it a valuable tool for data scientists.
Another benefit is the ability to make more accurate predictions. AI algorithms can learn from data and make predictions based on patterns they identify. When combined with statistical methods, which provide a solid foundation for understanding data, the result is more accurate and reliable predictions.
The integration of AI with statistical methods also allows for more efficient data analysis. AI can automate many of the tasks involved in data analysis, freeing up time for data scientists to focus on interpreting the results and making strategic decisions. This efficiency can lead to significant cost savings and improved business outcomes.
Challenges of Integrating AI with Statistical Methods
Despite the many benefits, integrating AI with statistical methods is not without its challenges. One of the biggest hurdles is the difference in the underlying philosophies of the two approaches. AI focuses on prediction and automation, while statistics emphasizes understanding and interpretation. This difference can lead to conflicts when trying to integrate the two.
Another challenge is the complexity of AI algorithms. These algorithms can be difficult to understand and interpret, making it challenging to integrate them with statistical methods. However, with careful planning and implementation, these challenges can be overcome.
Strategies for Successful Integration
Successfully integrating AI with statistical methods requires careful planning and implementation. One of the most important steps is to understand the strengths and weaknesses of both approaches. This understanding will allow you to leverage the strengths of each approach and mitigate their weaknesses.
Another important step is to ensure that your data is clean and well-prepared. AI algorithms rely on high-quality data to make accurate predictions. Therefore, it's crucial to invest time and resources in data cleaning and preparation.
Finally, it's important to continually monitor and evaluate your integration efforts. This will allow you to identify any issues early and make necessary adjustments.
Case Studies of Successful Integration
There are several examples of successful integration of AI with statistical methods. One example is in the field of healthcare, where AI and statistical methods are used to predict patient outcomes and improve patient care. Another example is in the field of finance, where AI and statistical methods are used to predict market trends and make investment decisions.
These case studies demonstrate the potential of integrating AI with statistical methods. They show that with careful planning and implementation, this integration can lead to significant benefits.
The Future of AI and Statistical Methods
The integration of AI with statistical methods is a rapidly evolving field. As technology continues to advance and more data becomes available, the potential for this integration will only increase.
One of the most exciting developments is the use of AI to automate statistical analysis. This could significantly speed up the analysis process and free up time for data scientists to focus on interpreting the results and making strategic decisions.
Another exciting development is the use of AI to improve the accuracy of statistical predictions. By learning from data and identifying patterns, AI could significantly improve the accuracy of statistical predictions.
Embracing the Fusion of AI and Statistics
The integration of AI with statistical methods offers significant benefits, including the ability to handle large datasets, make more accurate predictions, and conduct more efficient data analysis. Despite the challenges, with careful planning and implementation, this integration can lead to significant benefits. As technology continues to advance, the potential for this integration will only increase. Embracing this fusion of AI and statistics is not just an option, but a necessity for data scientists and statisticians looking to stay at the forefront of their field.