Latest Trends in Statistical Modeling
Statistician Zone
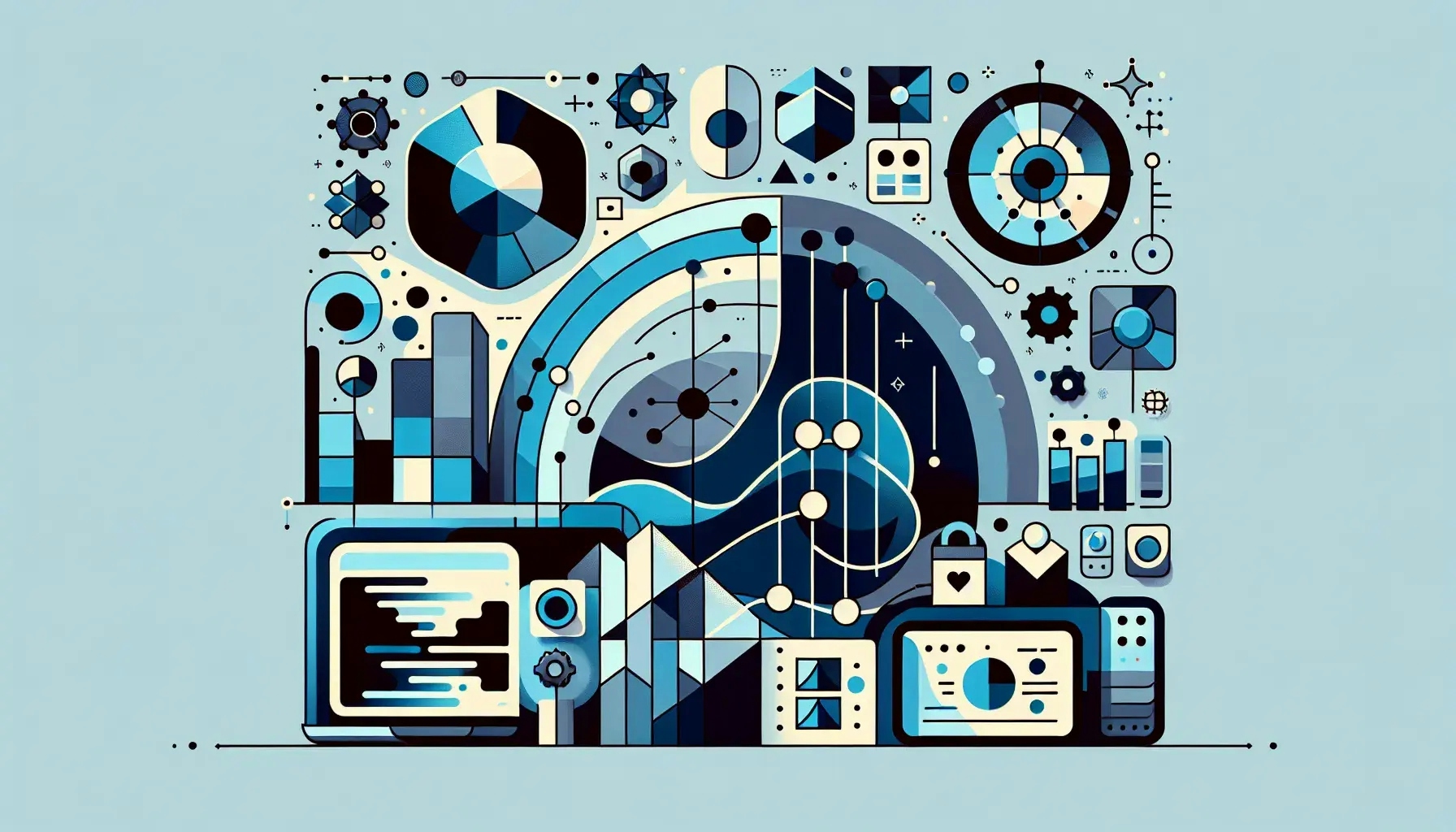
Welcome to the fascinating world of statistical modeling! This blog post aims to shed light on the latest trends that are shaping this dynamic field. As data continues to grow in volume and complexity, statistical modeling techniques are evolving to keep pace. From machine learning to Bayesian methods, we will delve into the most exciting developments that are pushing the boundaries of data analysis and interpretation.
The Rise of Machine Learning
Machine learning has taken the world of statistical modeling by storm. It's a trend that's impossible to ignore. Machine learning algorithms are now capable of analyzing vast amounts of data and making predictions with unprecedented accuracy.
The beauty of machine learning lies in its ability to learn from data without explicit programming. It's a shift away from traditional statistical models that relied heavily on predefined assumptions. Machine learning models can adapt to new data, improving their predictions over time.
One of the most popular techniques in machine learning is deep learning. Deep learning models, inspired by the human brain's neural networks, are capable of processing complex patterns in large data sets. They are particularly effective in areas such as image and speech recognition.
The Emergence of Bayesian Methods
Bayesian methods are another trend that's making waves in statistical modeling. These methods are based on Bayes' theorem, a fundamental principle in probability theory. Bayesian methods offer a flexible approach to modeling, allowing for the incorporation of prior knowledge into the analysis.
Unlike traditional statistical methods, Bayesian methods provide a full probability distribution of the parameters. This gives a more comprehensive view of uncertainty. Bayesian methods are also well-suited for dealing with complex hierarchical structures and missing data, which are common challenges in statistical modeling.
Bayesian methods are gaining traction in various fields, from healthcare to finance. They are being used to model complex phenomena and make predictions under uncertainty.
The Power of Big Data
Big data is reshaping the landscape of statistical modeling. With the explosion of data in today's digital age, statisticians are grappling with new challenges and opportunities.
Big data brings with it the promise of uncovering hidden patterns and insights. However, it also presents challenges in terms of storage, processing, and analysis. Traditional statistical methods often fall short when dealing with big data.
To harness the power of big data, new techniques and tools are emerging. These include distributed computing frameworks like Hadoop and Spark, and machine learning algorithms designed for big data. These tools are enabling statisticians to extract value from big data and drive decision-making.
The Impact of Artificial Intelligence
Artificial Intelligence (AI) is another trend that's influencing statistical modeling. AI, particularly machine learning, is being used to automate the process of building and refining models.
AI can sift through vast amounts of data, identify patterns, and generate predictive models. This can significantly speed up the modeling process and improve accuracy. AI is also being used to automate the selection of variables and the tuning of model parameters.
The integration of AI into statistical modeling is opening up new possibilities. It's enabling statisticians to tackle complex problems and generate insights faster than ever before.
The Shift Towards Real-Time Analytics
Real-time analytics is a trend that's gaining momentum in statistical modeling. In today's fast-paced world, the ability to analyze data in real-time is becoming increasingly important.
Real-time analytics allows for immediate interpretation of data as it's collected. This can provide a competitive edge in fields where timely decision-making is crucial. For instance, in finance, real-time analytics can be used to detect fraudulent transactions as they occur.
To support real-time analytics, new technologies and techniques are being developed. These include stream processing frameworks and online learning algorithms. These tools are enabling statisticians to deliver insights at the speed of business.
The Adoption of Open Source Tools
The use of open source tools is a trend that's transforming statistical modeling. Tools like R and Python have become staples in the toolkit of many statisticians.
Open source tools offer a number of advantages. They are free to use, highly customizable, and supported by a vibrant community of users. They also offer a wide range of packages for various statistical modeling techniques.
The adoption of open source tools is democratizing access to statistical modeling. It's enabling more people to harness the power of statistics and contribute to the field.
The Future of Statistical Modeling: A World of Possibilities
The world of statistical modeling is evolving at a rapid pace. The trends we've discussed - machine learning, Bayesian methods, big data, AI, real-time analytics, and open source tools - are reshaping the field. They are opening up new possibilities for data analysis and interpretation. As we move forward, it's clear that the future of statistical modeling holds exciting opportunities for those willing to embrace these trends.