Software Tools Preferred by Statisticians
Statistician Zone
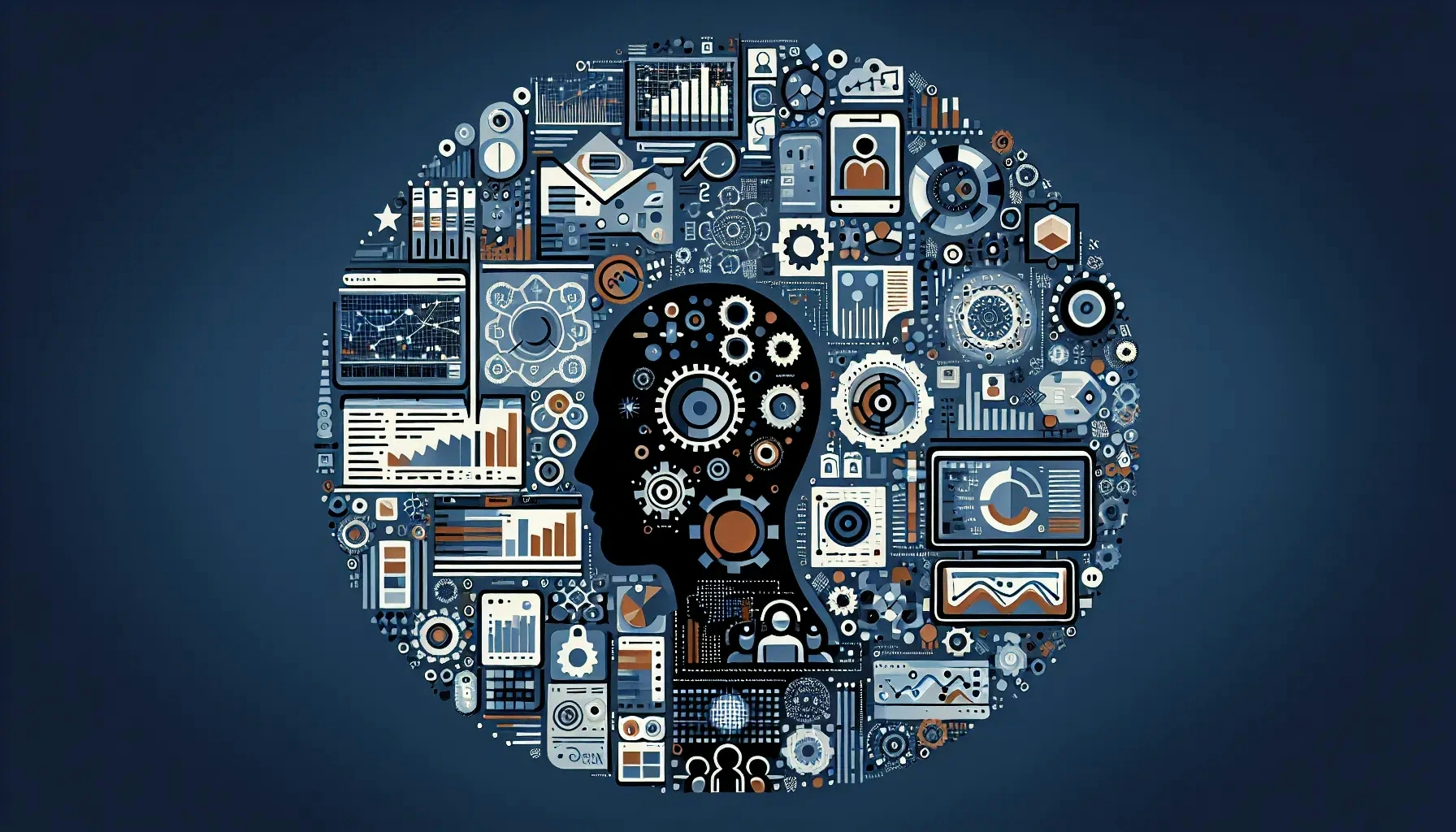
Welcome to our comprehensive exploration of the software tools that statisticians prefer. In this blog post, we will delve into the world of statistics, a field that heavily relies on specific software tools to collect, analyze, and interpret data. We will uncover the reasons why certain software tools stand out among the rest and how they cater to the unique needs of statisticians.
The Importance of Software Tools in Statistics
In the realm of statistics, software tools hold a pivotal role. They assist statisticians in managing and interpreting vast amounts of data. Without these tools, the process of data analysis could become overwhelming and time-consuming.
Statisticians use these tools to perform a variety of tasks. These tasks range from data collection and data cleaning to advanced statistical analysis and data visualization. Each task requires a tool that can handle its specific demands. Therefore, the choice of software tools often depends on the nature of the task at hand.
Moreover, the use of software tools in statistics is not merely a matter of convenience. These tools enable statisticians to perform complex analyses that would be nearly impossible to do manually. They also ensure the accuracy and reliability of the results, which is crucial in a field where precision is paramount.
Popular Software Tools Among Statisticians
Several software tools have gained popularity among statisticians due to their robust capabilities and user-friendly interfaces. Among these tools, R, Python, and SAS stand out.
R is a free software environment for statistical computing and graphics. It provides a wide array of statistical techniques such as linear and nonlinear modeling, classical statistical tests, time-series analysis, classification, and clustering. Its open-source nature allows statisticians to modify and improve it continuously, making it a versatile tool for various statistical tasks.
Python, on the other hand, is a high-level programming language that is known for its readability and simplicity. While not specifically designed for statistics, Python has numerous libraries like NumPy, SciPy, and pandas that make it suitable for statistical analysis. Its versatility and ease of learning make it a favorite among statisticians.
SAS (Statistical Analysis System) is a software suite developed by SAS Institute for advanced analytics, multivariate analyses, business intelligence, data management, and predictive analytics. It is particularly popular in the corporate world due to its robust data handling capabilities and extensive support for various types of analyses.
Factors Influencing the Choice of Software Tools
The choice of software tools among statisticians is influenced by several factors. These include the nature of the task, the complexity of the data, the required level of precision, and the user's familiarity with the tool.
The nature of the task is a crucial factor. Some tasks require specific statistical techniques, which are only available in certain software tools. For instance, if a task requires advanced multivariate analysis, a statistician might prefer SAS over other tools due to its extensive support for this type of analysis.
The complexity of the data also plays a significant role. Some software tools are better equipped to handle large and complex datasets than others. For example, R and Python are known for their ability to handle large datasets efficiently.
The required level of precision is another important factor. In tasks where precision is paramount, statisticians might prefer tools that provide more accurate and reliable results. For instance, SAS is known for its high level of precision, making it a preferred choice for tasks that require high accuracy.
Lastly, the user's familiarity with the tool can influence the choice. Statisticians tend to prefer tools that they are familiar with and comfortable using. This is because familiarity with the tool can enhance efficiency and productivity.
The Future of Software Tools in Statistics
The future of software tools in statistics looks promising. With the advent of big data and machine learning, the demand for advanced statistical tools is on the rise. These tools will need to handle larger datasets, perform more complex analyses, and provide more accurate results.
One trend that is likely to shape the future of statistical software tools is the integration of artificial intelligence (AI). AI can automate many statistical tasks, making the process of data analysis more efficient. It can also improve the accuracy of the results by learning from previous analyses and making predictions based on this learning.
Another trend is the development of more user-friendly interfaces. As the field of statistics becomes more accessible to non-statisticians, there is a growing demand for software tools that are easy to use. This will likely lead to the development of tools with more intuitive interfaces and simpler learning curves.
Challenges in the Use of Software Tools in Statistics
Despite the numerous advantages of using software tools in statistics, there are also challenges. One of the main challenges is the steep learning curve associated with some of these tools. Tools like R and SAS require a good understanding of programming, which can be daunting for beginners.
Another challenge is the cost. While some tools like R and Python are free, others like SAS can be quite expensive. This can be a barrier for individuals or organizations with limited resources.
Data security is another concern. With the increasing use of cloud-based tools, there is a risk of data breaches. Therefore, it is crucial for software tools to have robust security measures in place to protect the data.
Overcoming Challenges in the Use of Software Tools in Statistics
Despite the challenges, there are ways to overcome them. For the steep learning curve, there are numerous resources available online to help beginners learn these tools. These include tutorials, online courses, and forums where users can ask questions and get help.
For the cost, one solution is to use free alternatives. Tools like R and Python are not only free but also offer a wide range of capabilities that can rival those of paid tools. For organizations, investing in training for these free tools can be a cost-effective solution.
For data security, it is important to choose tools that prioritize data protection. This includes tools with strong encryption, user authentication, and regular security updates. It is also crucial to follow best practices for data security, such as not sharing sensitive data and using strong passwords.
Wrapping Up: Preferred Software Tools Among Statisticians
In conclusion, software tools play a crucial role in the field of statistics. They assist statisticians in managing and interpreting vast amounts of data, making their work more efficient and accurate. While the choice of tools can vary depending on several factors, R, Python, and SAS stand out as the most preferred. Despite the challenges associated with these tools, there are ways to overcome them, ensuring that statisticians can continue to leverage these tools to their advantage.