Understanding Statistical Significance
Statistician Zone
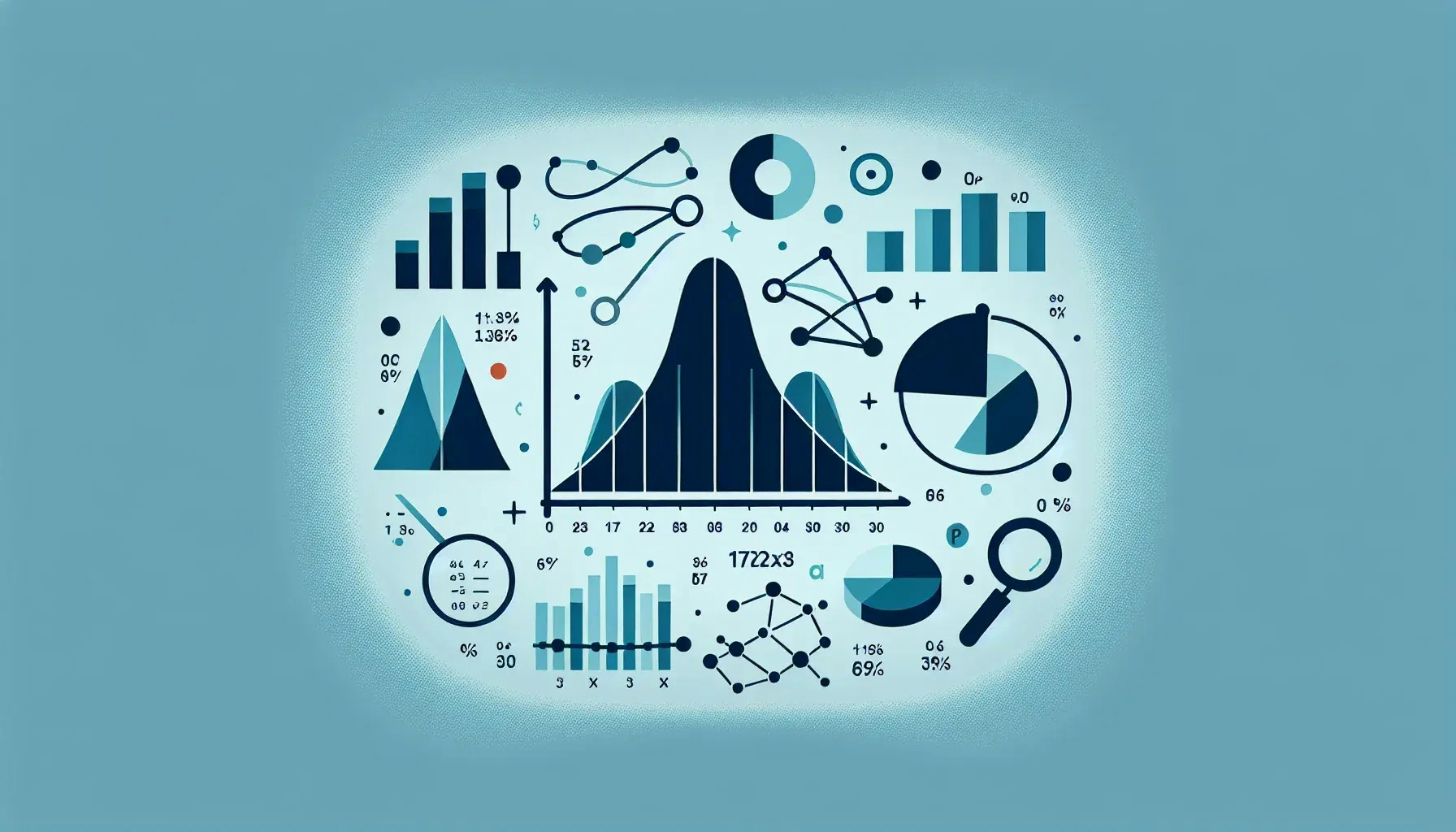
Welcome to this comprehensive guide on understanding statistical significance. This concept is a cornerstone of statistical analysis, often used in scientific research, business decisions, and policy-making. It helps us determine whether the results of a study or an experiment are due to chance or if they reflect an actual trend. In this blog post, we will delve into the depths of statistical significance, its importance, and how to interpret it.
The Basics of Statistical Significance
Let's start with the basics. Statistical significance is a term used in hypothesis testing to help us understand if an observed effect in our data is real or if it's likely due to chance. It's a tool that researchers use to confirm or refute their hypotheses.
Statistical significance is determined by a number called the p-value. The p-value is a probability that measures the evidence against the null hypothesis. The null hypothesis, in simple terms, is a statement that there is no effect or relationship in the population. If the p-value is small, typically less than 0.05, we reject the null hypothesis and conclude that the observed effect is statistically significant.
However, it's important to note that statistical significance does not necessarily mean practical significance. An effect might be statistically significant but may not have a meaningful impact in the real world. For instance, a drug might significantly reduce the risk of a disease, but the reduction might be so small that it doesn't make a difference in practice.
Understanding the P-value
The p-value is a crucial part of understanding statistical significance. It's a probability that ranges from 0 to 1. A smaller p-value indicates stronger evidence against the null hypothesis.
Let's say we have a p-value of 0.03. This means there's a 3% chance that the observed effect occurred by chance, assuming the null hypothesis is true. If we set our significance level at 0.05, this p-value would lead us to reject the null hypothesis and conclude that the effect is statistically significant.
However, the p-value is often misunderstood. It's not the probability that the null hypothesis is true. It's also not the probability that our results occurred by chance alone. Rather, it's the probability of observing our results, or more extreme results, assuming the null hypothesis is true.
The Role of Sample Size in Statistical Significance
Sample size plays a critical role in determining statistical significance. A larger sample size can make an effect more statistically significant, even if the effect is small.
This is because a larger sample size reduces the standard error, which is a measure of the variability in our estimate. A smaller standard error means our estimate is more precise, increasing the chances of finding a statistically significant effect.
However, a large sample size can also lead to problems. It can make small, unimportant effects statistically significant. This is why it's crucial to consider the practical significance of an effect, not just its statistical significance.
Confidence Intervals and Statistical Significance
Confidence intervals provide another way to understand statistical significance. A confidence interval is a range of values that likely contains the true population parameter.
If a 95% confidence interval for a mean difference does not include zero, we can conclude that the mean difference is statistically significant at the 0.05 level. This is because the confidence interval is the range of values that we are 95% confident contains the true population mean difference. If this range does not include zero, it means that we are 95% confident that there is a real difference in the population.
Confidence intervals give us more information than the p-value alone. They provide a range of plausible values for the population parameter and give us an idea of the precision of our estimate.
Misconceptions and Misuses of Statistical Significance
Despite its widespread use, statistical significance is often misunderstood and misused. One common misconception is that statistical significance means practical significance. As we've discussed, this is not the case. A statistically significant effect might be small and unimportant in practice.
Another common misuse of statistical significance is the so-called "p-hacking". This is the practice of performing many tests until a statistically significant result is found. This increases the likelihood of finding a significant result by chance alone and is a form of bias.
It's also important to remember that statistical significance does not tell us about the size of an effect. An effect might be statistically significant, but it might be so small that it's not meaningful in practice.
The Importance of Understanding Statistical Significance
Understanding statistical significance is crucial in many fields. In scientific research, it helps us determine whether the results of a study are due to chance or reflect a real effect. In business, it can help us make data-driven decisions. In policy-making, it can help us evaluate the effectiveness of policies.
However, it's important to use statistical significance correctly and understand its limitations. It's a tool, not a definitive answer. It should be used in conjunction with other statistical measures and practical considerations.
Wrapping Up: The Journey to Understanding Statistical Significance
We've embarked on a comprehensive journey to understand statistical significance. We've explored its basics, delved into the concept of the p-value, discussed the role of sample size, and looked at confidence intervals. We've also addressed common misconceptions and misuses of statistical significance. Remember, statistical significance is a tool to help us make sense of our data. It's not an end in itself, but a means to an end. It's our responsibility to use it wisely and interpret it correctly.