6 Misconceptions About Statistics and How to Address Them
Statistics play a crucial role in shaping our understanding of the world, yet they are often misunderstood or misinterpreted. Common misconceptions about statistical concepts can lead to flawed conclusions and decision-making in various fields, from scientific research to business analytics. This article aims to shed light on six key misconceptions in statistics, providing clarity on topics such as correlation, causation, and the importance of sample size.
- Correlation Does Not Imply Causation
- P-Values Misinterpreted in Hypothesis Testing
- Sample Size Affects Statistical Significance
- Confounding Variables Skew Data Interpretation
- Averages Can Mask Important Data Distributions
- Effect Size Crucial Beyond Statistical Significance
Correlation Does Not Imply Causation
One common misconception I encounter frequently is that correlation equals causation—people observe two things moving together and immediately assume one causes the other.
For example, I once had a client who believed a spike in blog traffic caused their sales to drop because the timelines coincided. In reality, the traffic was unrelated—it was a seasonal spike from a non-buyer audience, and their sales dip was due to a supply chain issue!
How I address it:
I always break it down visually. I show a simple chart with two unrelated trends (like ice cream sales and shark attacks) that rise together in summer. It makes people laugh, but it drives home the point: just because two things happen simultaneously doesn't mean they're connected.
What's important to understand:
Statistics are powerful, but they need context and critical thinking. Always ask: "Is there a logical mechanism that connects these two things?" If not, dig deeper before making decisions based on the data.
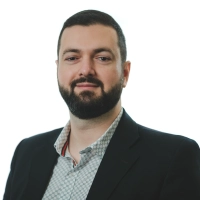
P-Values Misinterpreted in Hypothesis Testing
P-values are often misunderstood in hypothesis testing, leading to incorrect conclusions. Many researchers mistakenly believe that a low p-value proves their hypothesis is true. However, p-values only indicate the likelihood of obtaining the observed results if the null hypothesis were true. This misconception can result in overconfidence in research findings and the publication of false-positive results.
Understanding the true meaning of p-values is crucial for accurate statistical interpretation. Researchers should focus on effect sizes and confidence intervals alongside p-values for a more comprehensive analysis. Take time to learn the proper interpretation of p-values to improve the quality of statistical research.
Sample Size Affects Statistical Significance
The impact of sample size on statistical significance is frequently underestimated in research. A larger sample size can lead to statistically significant results even when the effect size is small and practically meaningless. Conversely, small sample sizes may fail to detect important effects due to lack of statistical power. This misconception can result in overconfidence in studies with large samples or dismissal of potentially important findings in smaller studies.
Researchers should consider both statistical significance and effect size when interpreting results. It's important to determine appropriate sample sizes through power analysis before conducting studies. Always evaluate the practical significance of results, regardless of sample size.
Confounding Variables Skew Data Interpretation
Overlooking confounding variables is a common pitfall in statistical analysis that can lead to flawed interpretations. Confounding variables are factors that influence both the independent and dependent variables, potentially creating a false association between them. Failing to account for these variables can result in incorrect conclusions about cause-and-effect relationships. This oversight may lead to the implementation of ineffective policies or treatments based on misleading data.
Researchers must carefully consider potential confounding factors in their study design and analysis. It's crucial to use appropriate statistical techniques, such as multiple regression or propensity score matching, to control for confounding variables. Make sure to thoroughly examine all possible influencing factors before drawing conclusions from statistical analyses.
Averages Can Mask Important Data Distributions
The misuse of averages in statistical reporting often obscures important information about data distribution. While averages provide a simple summary, they can be misleading when the data is skewed or contains outliers. This can lead to inaccurate interpretations and poor decision-making based on incomplete information. For example, using average income to represent a population's economic status may hide significant wealth disparities.
It's important to consider other measures of central tendency and dispersion, such as median and standard deviation, alongside averages. Researchers should also use visual representations like histograms or box plots to better understand data distribution. Always look beyond averages to gain a more comprehensive understanding of the data at hand.
Effect Size Crucial Beyond Statistical Significance
Neglecting effect size while focusing solely on statistical significance is a common error in statistical interpretation. Statistical significance indicates whether an observed difference is likely due to chance, but it doesn't reveal the magnitude or practical importance of that difference. This oversight can lead to overemphasis on trivial findings or underappreciation of meaningful results that lack statistical significance due to small sample sizes.
Effect size measures, such as Cohen's d or correlation coefficients, provide crucial information about the strength and practical significance of relationships or differences. Researchers should report and interpret effect sizes alongside p-values in their analyses. Remember to consider both statistical and practical significance when evaluating research findings to make well-informed decisions.