6 Versatile Statistical Tests Used Across Disciplines
Statistical tests are the backbone of data analysis across various fields of study. From comparing group means to analyzing categorical data, these powerful tools help researchers draw meaningful conclusions from their datasets. This blog post explores six versatile statistical tests that are widely used in different disciplines, providing insights into their applications and significance.
- Chi-Square Test: Versatile Categorical Analysis Tool
- ANOVA: Comparing Means Across Multiple Groups
- T-Test: Evaluating Differences Between Two Populations
- Regression Analysis: Predicting Outcomes and Relationships
- Mann-Whitney U: Non-Parametric Alternative to T-Test
- Kruskal-Wallis: Non-Parametric Test for Multiple Groups
Chi-Square Test: Versatile Categorical Analysis Tool
One statistical test that has proven indispensable in my toolbox is the chi-square test of independence. This test is incredibly versatile because it helps determine whether there's a significant association between two categorical variables. For example, it's excellent for analyzing marketing data to see if the preference for a product varies by demographic categories like age or gender. It can also be used in healthcare to examine the relationship between treatment types and patient outcomes, providing insights that are critical for improving service delivery.
What makes the chi-square test particularly useful is its applicability across different fields without requiring assumptions about the distribution of data, which is a common prerequisite in other statistical tests. Whether I'm working with customer feedback, medical records, or survey responses, the chi-square test provides valuable insights that help drive strategic decisions and innovations. It's a straightforward tool, but the depth of understanding it provides into the connections between variables is invaluable, making it a go-to method in diverse research scenarios.
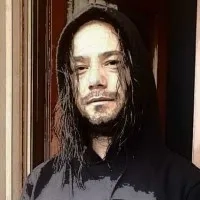
ANOVA: Comparing Means Across Multiple Groups
ANOVA, or Analysis of Variance, is a powerful statistical test used to compare means across multiple groups. This versatile method allows researchers to determine if there are significant differences between three or more groups. ANOVA is widely applied in fields such as psychology, biology, and marketing to analyze experimental results.
It helps in understanding how different factors influence a specific outcome. By using ANOVA, researchers can make informed decisions about their hypotheses and draw meaningful conclusions from their data. Consider using ANOVA in your next research project to gain valuable insights into group differences.
T-Test: Evaluating Differences Between Two Populations
The t-test is a fundamental statistical tool used to evaluate differences between two populations. This test is particularly useful when working with small sample sizes and when the population standard deviation is unknown. Researchers often employ t-tests in medical studies, social sciences, and quality control processes.
The t-test helps determine if the differences observed between two groups are statistically significant or merely due to chance. Understanding and applying t-tests can greatly enhance the validity of research findings. Take the time to learn more about t-tests and how they can strengthen your analytical skills.
Regression Analysis: Predicting Outcomes and Relationships
Regression analysis is a vital statistical method used to examine relationships between dependent and independent variables. This technique allows researchers to predict outcomes based on one or more predictor variables. Regression is widely used in economics, finance, and environmental sciences to model complex relationships.
It helps in understanding how changes in one variable affect another, which is crucial for decision-making and policy formulation. Regression analysis can reveal patterns and trends that might not be immediately apparent in raw data. Explore regression techniques to uncover hidden insights in your data and make more informed predictions.
Mann-Whitney U: Non-Parametric Alternative to T-Test
The Mann-Whitney U test is a non-parametric alternative to the t-test, used when data doesn't meet the assumptions of normality. This test is particularly useful in social sciences, ecology, and medical research where ordinal data is common. The Mann-Whitney U test compares two groups to determine if one group tends to have higher values than the other.
It's especially valuable when dealing with small sample sizes or when data is not normally distributed. This test provides a robust way to analyze data that doesn't fit traditional parametric methods. Consider using the Mann-Whitney U test in your next project involving ordinal or non-normal data.
Kruskal-Wallis: Non-Parametric Test for Multiple Groups
The Kruskal-Wallis test is a non-parametric version of one-way ANOVA, used when assumptions of normality are not met. This test is particularly useful in fields like ecology, social sciences, and medical research where data often doesn't follow a normal distribution. The Kruskal-Wallis test allows researchers to compare three or more groups to determine if they come from the same distribution.
It's especially valuable when dealing with ordinal data or when sample sizes are small. This test provides a way to analyze data that doesn't fit the requirements for parametric tests. Explore the Kruskal-Wallis test to expand your statistical toolkit for analyzing non-normal data across multiple groups.