7 Tips for Interpreting Statistical Analysis Results
Statistical analysis can be a powerful tool, but interpreting the results correctly is crucial for making informed decisions. This article presents expert-backed tips to help readers navigate the complexities of statistical interpretation. By focusing on key aspects such as context, effect size, and real-world relevance, readers will gain valuable insights into extracting meaningful information from statistical data.
- Start with the Question, Not the Numbers
- Focus on Context and Key Elements
- Consider Effect Size, Not Just Significance
- Evaluate Confounding Variables and Study Limitations
- Understand the Impact of Research Design
- Assess Real-World Relevance of Results
- Compare Findings with Existing Knowledge
Start with the Question, Not the Numbers
My advice: Don't rush to a conclusion; start with the question itself. If the analysis doesn't answer what you set out to understand, go back. Recheck assumptions, such as the sample size, data quality, and whether the model fits the story or distorts it. Often, statistical significance is not found in insight but in practical meaning. A tiny p-value means little if the effect is trivial. Always ask: Could this result mislead someone? If yes, then dig deeper. Interpretation is less about math and more about judgment.
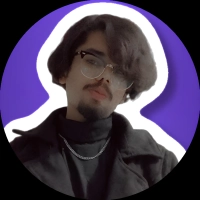
Focus on Context and Key Elements
If you're struggling to interpret the results of a statistical analysis, my advice would be to focus on the context and the story the data is telling rather than getting lost in the numbers themselves. Start by understanding what the analysis is trying to measure. Are you looking for correlations, differences between groups, or something else? Once you have that clarity, pay attention to a few key elements:
First, check the significance level (p-value). A commonly accepted threshold is 0.05, which indicates whether the results are likely due to chance. However, it's important to also consider the effect size, which tells you the practical significance of the result—not just if it's statistically significant but whether it actually matters.
Second, look at the sample size. A small sample size might produce misleading results, while a larger sample can give you more reliable insights. Also, check for any biases in the data collection process.
Finally, visualize the results if you can. Sometimes, data can speak more clearly through a graph or chart, helping you see patterns or trends that are hard to interpret from numbers alone.
By focusing on these key points, you'll have a much better understanding of what the analysis means and how it applies to the real world.
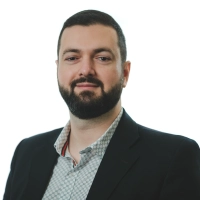
Consider Effect Size, Not Just Significance
When interpreting statistical analysis results, it's crucial to look beyond just statistical significance and consider the effect size. Statistical significance only tells us if a result is likely due to chance, while effect size reveals the magnitude of the relationship or difference. A small effect might be statistically significant in a large sample, but it may not be practically important. Researchers should always report both statistical significance and effect size measures.
This combination provides a more complete picture of the findings. By understanding both aspects, decision-makers can better judge the real-world impact of the results. Take the time to learn about different effect size measures and their interpretations to enhance your statistical analysis skills.
Evaluate Confounding Variables and Study Limitations
Evaluating potential confounding variables and limitations is a critical step in interpreting statistical analysis results. Confounding variables are factors that might influence both the independent and dependent variables, potentially leading to misleading conclusions. Researchers must identify and control for these variables to ensure the validity of their findings. Additionally, every study has limitations that could affect the interpretation of results. These might include sample size, data collection methods, or generalizability issues.
Understanding these limitations helps in accurately contextualizing the results. It's important to critically examine how confounders and limitations might have influenced the outcomes. By doing so, one can avoid overstating conclusions or misinterpreting the data. Make it a habit to thoroughly review the methods section and limitations discussion in research papers to gain a more nuanced understanding of the results.
Understand the Impact of Research Design
Examining a study's methodology and design is essential for properly interpreting statistical analysis results. The chosen research design, whether experimental, observational, or correlational, significantly impacts the types of conclusions that can be drawn. For instance, only experimental designs can establish causality, while correlational studies can only show relationships. The sampling method used affects how well the results can be generalized to larger populations.
Data collection techniques and measurement tools influence the reliability and validity of the data. Understanding these methodological aspects helps in assessing the strength of the evidence presented. It also aids in identifying potential sources of bias or error in the study. Take the initiative to familiarize yourself with different research designs and their implications to become a more discerning consumer of statistical analyses.
Assess Real-World Relevance of Results
Assessing the relevance of statistical analysis results to real-world applications is a crucial step in interpretation. While a study might show statistically significant results, it's important to consider whether these findings have practical significance in everyday contexts. This involves thinking about how the results might translate into tangible outcomes or policy changes. The magnitude of effects should be considered in light of real-world constraints and opportunities.
It's also important to think about the specific context in which the study was conducted and how this might differ from other settings. Researchers and practitioners should carefully consider the potential impacts and limitations of applying the results in various situations. Strive to bridge the gap between academic findings and practical applications by always asking how the results could be meaningfully implemented in real-world scenarios.
Compare Findings with Existing Knowledge
Comparing statistical analysis results with existing literature and theories is an important part of the interpretation process. No study exists in isolation, and new findings should be considered within the broader context of established knowledge. This comparison can reveal whether the results support, contradict, or extend current understanding in the field. Consistency with previous findings can increase confidence in the results, while contradictions may highlight areas needing further investigation.
It's also important to consider how well the results align with theoretical predictions. This process of situating new findings within the existing body of knowledge helps in building a more comprehensive understanding of the topic. Make an effort to conduct thorough literature reviews and stay updated with current theories in your field to enhance your ability to contextualize and interpret new statistical results.