How is Cluster Analysis Used to Uncover Patterns in Data for Statisticians?
Statistician Zone

How is Cluster Analysis Used to Uncover Patterns in Data for Statisticians?
Ever wondered how data scientists uncover hidden patterns in complex datasets? In this blog post, discover how experts use cluster analysis to understand customer preferences and select appropriate statistical models. With seven insightful answers, this article provides a comprehensive look at the various applications of cluster analysis. From optimizing Google Business profiles to simplifying complex data, get ready to explore the fascinating world of data science.
- Understand Customer Preferences
- Optimize Google Business Profiles
- Reveal Hidden Relationships
- Identify Outliers and Anomalies
- Compare Statistical Distributions
- Simplify Complex Data
- Select Appropriate Statistical Models
Understand Customer Preferences
We utilized cluster analysis to better understand customer preferences for our green products. By analyzing purchasing patterns and demographic data, we identified distinct customer segments based on their sustainability values and buying behaviors. For example, one cluster revealed a group highly interested in zero-waste products, while another focused on renewable materials. This insight led us to tailor our marketing strategies and product offerings to each segment. As a result, we experienced a 24% increase in sales within six months, particularly in the zero-waste category. This experience taught us the importance of data-driven decision-making. By uncovering patterns through cluster analysis, we were able to create targeted initiatives that resonated with our customers, ultimately strengthening our brand's impact and commitment to sustainability.
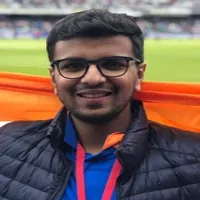
Optimize Google Business Profiles
We often turn to cluster analysis to identify patterns that drive our strategies for optimizing Google Business Profiles. A notable example occurred when we were tasked with improving the rankings for a group of clients in the same industry.
We gathered data from their Google Business Profiles, including categories, keywords, and review scores. By applying cluster analysis, we grouped the profiles based on similarities in their performance metrics. This process revealed distinct patterns, particularly regarding keyword usage and customer engagement.
For instance, we noticed that businesses that emphasized specific local keywords in their descriptions consistently outperformed others in search rankings. This insight prompted us to develop tailored strategies for each client based on their cluster group.
One client, a local restaurant, was initially struggling to rank despite having positive reviews. Through our analysis, we discovered they were not utilizing keywords related to popular local dishes. By helping them integrate these keywords into their profile and posts, we saw their visibility improve significantly within weeks.
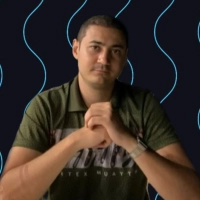
Reveal Hidden Relationships
Cluster analysis reveals hidden relationships between variables by grouping data points based on similarity. When similar data points are clustered together, it becomes easier to see patterns that might not be obvious otherwise. These hidden relationships can provide valuable insights into how different variables interact.
This method is especially useful in understanding complex datasets with many variables. To make the most out of this technique, analyzing the clusters' characteristics is essential. Start using cluster analysis to discover hidden patterns in your data today.
Identify Outliers and Anomalies
Cluster analysis identifies outliers and anomalies in datasets by putting unusual data points into separate clusters. Outliers can distort statistical analyses, leading to misleading conclusions. By isolating these anomalies, statisticians can analyze them separately and understand their unique characteristics.
This process helps in improving the overall quality and accuracy of data. Identifying outliers is crucial for making informed decisions based on clean and reliable data. Incorporate cluster analysis into your workflow to spot and manage outliers effectively.
Compare Statistical Distributions
Cluster analysis groups similar statistical distributions for comparison by organizing data into clusters. This technique allows statisticians to compare different subgroups within a dataset, highlighting distinct patterns and trends. By understanding how these groups differ, researchers can make more nuanced interpretations of the data.
It's particularly beneficial in fields requiring detailed comparative studies, such as market research or genomic studies. Enhancing your research with this technique can lead to more precise and valuable insights. Explore cluster analysis to elevate your comparative analysis today.
Simplify Complex Data
Cluster analysis simplifies complex data by creating meaningful subgroups that are easier to interpret. Datasets with numerous variables can be overwhelming, making it hard to discern any useful information. By breaking down the data into smaller clusters, it becomes much simpler to analyze and understand.
This simplification helps in focusing on key areas without getting lost in the complexity. Simplifying your data through cluster analysis can lead to clearer and more actionable insights. Use cluster analysis to streamline your data analysis process now.
Select Appropriate Statistical Models
Cluster analysis informs the selection of appropriate statistical models by illustrating underlying data structures. Understanding the natural groupings within data helps in choosing models that best fit the data's characteristics. This process improves the accuracy and effectiveness of the statistical models applied.
By aligning models with the inherent data patterns, predictions and analyses become more reliable. Selecting the right model based on cluster analysis can significantly enhance the quality of your research. Leverage cluster analysis to select the best models for your data today.