What Approaches Do You Take to Quantify Uncertainty in Predictions?
Statistician Zone

What Approaches Do You Take to Quantify Uncertainty in Predictions?
In the quest to quantify uncertainty within predictive models, we've gathered insights starting with a Data Scientist's perspective on embracing ensemble methods. Alongside expert opinions, we've included additional approaches to provide a broader understanding of the strategies employed in different sectors. From sophisticated Monte Carlo simulations to the refinement of forecasts with Markov Chain models, explore the diverse methodologies professionals use to tackle uncertainty in their predictions.
- Embrace Ensemble Methods
- Introduce Monte Carlo Simulations
- Update Predictions with Bayesian Inference
- Interpret with Confidence Intervals
- Estimate Variability Using Bootstrapping
- Forecast with Markov Chain Models
- Enhance Precision with Variance Reduction
Embrace Ensemble Methods
We typically tend to use Bayesian inferences or evaluation metrics to combat uncertainty, but my personal favorite is Ensemble Methods, i.e., training multiple models with different architectures or hyperparameters and combining their predictions. Most of the time, this 'wisdom of the crowds' approach reduces overall uncertainty compared to a single model.
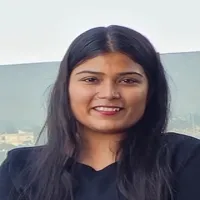
Introduce Monte Carlo Simulations
One approach I've taken to quantify uncertainty in my predictions is by using a technique called Monte Carlo simulations. Essentially, this involves running multiple simulations with a variety of input variables to see a range of possible outcomes. It's a bit like rolling the dice a thousand times to see all the different ways they might land.
I first introduced this method to our team when we were dealing with some high-stakes projections for a major condo development project. Initially, there was some skepticism—especially from those who were used to more straightforward, linear prediction models. However, once I demonstrated how it allowed us to see not just a single outcome but a spectrum of possibilities, the team began to appreciate its value. They saw that it provided a more comprehensive picture of risk and uncertainty, which is crucial in the real estate market where so many variables are at play.
Our clients also responded positively. They appreciated the transparency and felt more confident in our projections, knowing we had accounted for various scenarios. It really helped in building trust and setting realistic expectations.
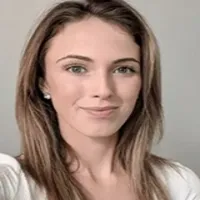
Update Predictions with Bayesian Inference
Bayesian inference serves as a powerful statistical approach where probability distributions are assigned to uncertain parameters. This method allows statisticians to update these probabilities as new data becomes available, essentially 'learning' from the data. It incorporates prior knowledge with new information to refine predictions.
Such a dynamic framework is particularly useful in complex systems where initial assumptions may evolve over time. Interested in handling uncertainty with a principled approach? Consider exploring Bayesian inference techniques in your next project.
Interpret with Confidence Intervals
Confidence intervals are utilized by statisticians to interpret the reliability of their results. Instead of providing a single-point estimate, confidence intervals offer a range within which the true value is likely to fall, which directly addresses uncertainty. Typically expressed in percentage terms, these intervals quantify the level of confidence that can be placed in the prediction.
This clarity is essential when making decisions based on statistical findings. If you're dealing with statistical results, ensure to ascertain the confidence intervals to better understand the potential variability.
Estimate Variability Using Bootstrapping
Bootstrapping is a resampling technique statisticians employ to estimate the uncertainty in their predictions. By repeatedly sampling from the data set with replacement, they create many 'bootstrap' samples upon which calculations can be performed. This process generates an empirical distribution of the estimator, giving insight into its variability.
It is as if one were able to redraw from the population repeatedly, without requiring the impossible task of collecting new samples each time. If uncertainty estimation poses a challenge in your analysis, try implementing bootstrapping to gain deeper insights.
Forecast with Markov Chain Models
Markov Chain models are applied by statisticians when predicting sequences that involve random processes. These models assume that the future state depends only on the present state and not on the sequence of events that preceded it. This 'memoryless' property simplifies complex stochastic processes and allows for the modeling of future events.
Markov Chain models are specifically useful in areas like economics, genetics, and game theory where one needs to forecast the behavior of dynamic systems. Investigate Markov Chain models to better forecast sequences with inherent randomness.
Enhance Precision with Variance Reduction
Variance reduction techniques are employed by statisticians aiming to enhance the precision of their predictions. These techniques involve methods that either simplify the underlying mathematical model or make the computational algorithm more efficient. The result is a more stable prediction with narrower confidence bounds, making the interpretation of results clearer.
This improvement in accuracy is crucial in fields where precision is paramount, like finance and engineering. When precision is key in your statistical analysis, consider applying variance reduction techniques to achieve a higher level of certainty.