What Are Common Data Myths Or Misconceptions You’ve Encountered?
Statistician Zone

What Are Common Data Myths Or Misconceptions You’ve Encountered?
Information is power, but what if it's based on misconceptions? A CEO shares their thoughts on the importance of data quality over quantity. Meanwhile, the final perspective discusses the necessity of considering the practical significance of results. Here are five expert insights guaranteed to reshape any organization's data strategy.
- Focus on Data Quality Over Quantity
- Distinguish Between Correlation and Causation
- Scrutinize Data Sources Carefully
- Acknowledge Biases in Data Collection
- Consider Practical Significance of Results
Focus on Data Quality Over Quantity
One instance where I had to debunk a data myth within our organization involved the misconception that a higher volume of data automatically leads to better insights. Some team members believed that collecting more data—regardless of quality—would provide more accurate or useful conclusions.
However, I explained that quality is more important than quantity. We were seeing issues with analysis because much of the data was either irrelevant or poorly structured, leading to inaccurate insights and decision-making. I showed that without proper data cleaning, relevance checks, and meaningful segmentation, even a large dataset can produce misleading or unreliable conclusions.
To address this, we shifted our focus to gathering high-quality, relevant data and emphasized the importance of proper analysis techniques, such as ensuring the data was aligned with our goals and properly verified before use. This change resulted in more actionable insights and improved decision-making, proving that it’s not the amount of data but its accuracy and relevance that truly matters.
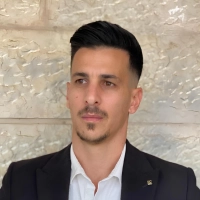
Distinguish Between Correlation and Causation
A common data myth statisticians encounter is the belief that correlation implies causation. This misconception arises when people see two variables moving together and assume one causes the other. However, both variables could be influenced by a third, unknown factor, or the relationship could be coincidental.
Incorrectly assuming causation can lead to faulty conclusions and misguided actions. It is crucial to conduct thorough research to explore possible confounding variables. Think critically and question whether there's really a cause-and-effect relationship at play.
Scrutinize Data Sources Carefully
Another misconception is that having more data will always lead to better, more accurate results. While it is true that larger datasets can reduce random errors, they can also magnify systematic biases if the data itself is flawed. Simply gathering more data without considering its quality can result in complex datasets that are difficult to interpret.
High-quality, relevant data is more important than just having a large quantity. Scrutinize your data sources carefully before expanding your dataset.
Acknowledge Biases in Data Collection
People often mistakenly believe that data itself is always objective and unbiased. However, data collection processes, measurement tools, and the people involved all have their own biases and limitations. These human factors can inadvertently shape the data, leading to skewed results.
Blindly relying on data without considering these biases can lead to significant errors. Always evaluate the context and methodology behind your data.
Consider Practical Significance of Results
Many think that statistical significance automatically means the result is practically important. Statistical significance simply shows that an observed effect is unlikely due to chance, but it doesn't tell you how meaningful or useful the result is in the real world. A statistically significant finding in a large sample might have a tiny effect that isn't important in practical terms.
It is essential to consider both statistical and practical significance when interpreting results. Make sure to assess the real-world relevance of your findings.