What Are Methods for Adapting Traditional Statistical Techniques for Modern Projects?
Statistician Zone
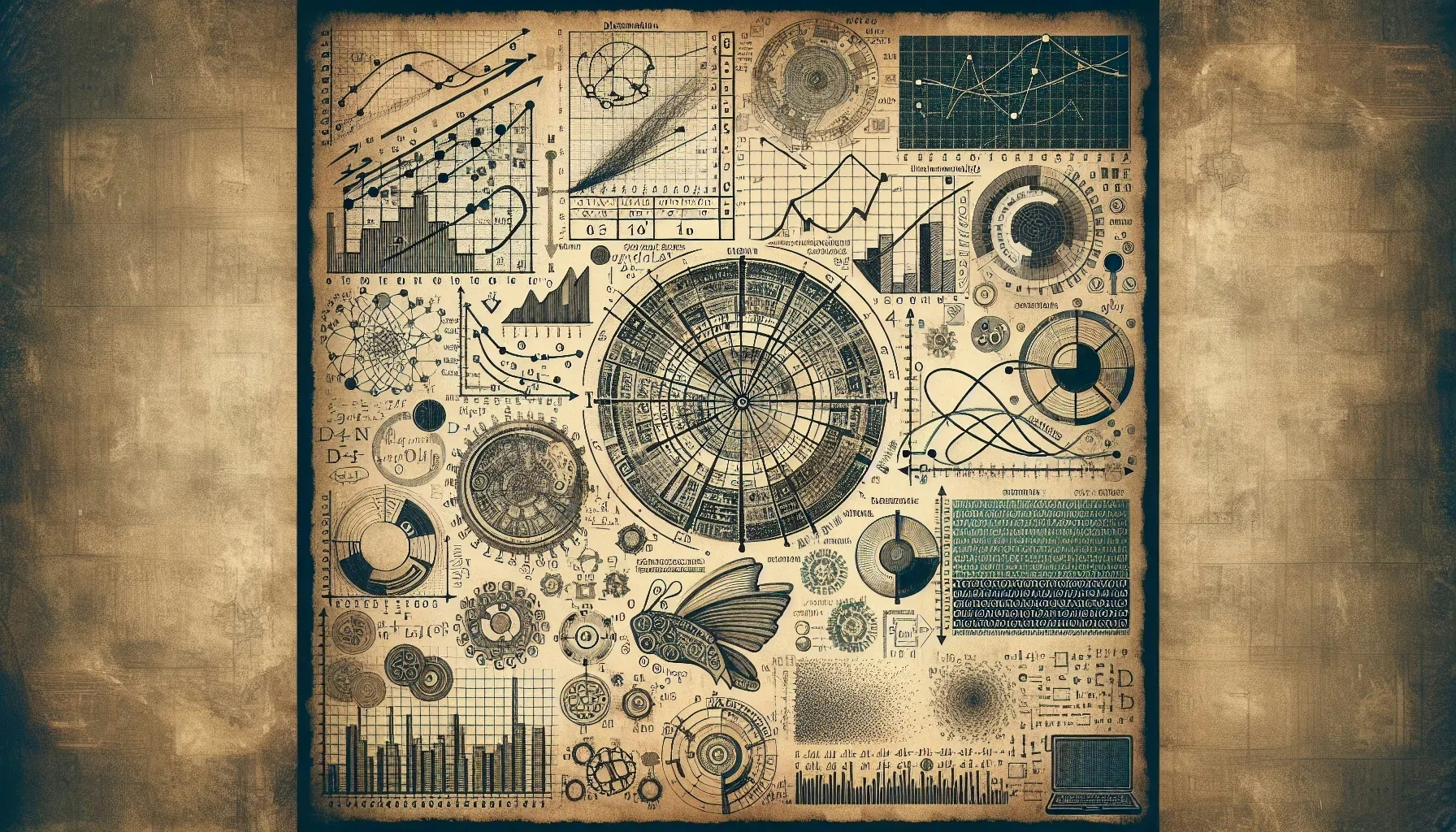
What Are Methods for Adapting Traditional Statistical Techniques for Modern Projects?
In the quest to modernize traditional statistical methods for today's data-driven projects, insights from a Real Estate Sales Representative & Montreal Division Manager reveal a creative blend of classic techniques with machine learning. Alongside expert perspectives, we've gathered additional answers that reflect the innovative adaptations being made in the field. From leveraging distributed computing to adopting regularization techniques, explore six ideas for reshaping statistical analysis to meet contemporary demands.
- Blend Classic and Machine Learning
- Integrate Real-Time Data Analysis
- Apply Robust Data Normalization
- Utilize Distributed Computing
- Incorporate Bayesian Methods
- Adopt Regularization Techniques
Blend Classic and Machine Learning
Back in the day, we relied heavily on classic regression analysis to make sense of market trends and forecast prices. But as the industry embraced the digital age and drowned in data, we knew we had to shake things up a bit.
We took a page out of the modern playbook and infused some machine-learning magic into our methodology. Instead of sticking solely to regression analysis, we started dabbling in random forests, neural networks, you name it. We wanted to squeeze every drop of insight from the vast sea of data at our disposal.
But here's where it gets interesting—we didn't abandon our old statistical methods. No way. We saw them as the foundation, the bedrock of our analysis. So, we took a more nuanced approach, blending the best of both worlds. We used regression analysis to pinpoint the key variables driving our models and then let the machine-learning algorithms work their magic, fine-tuning them to perfection.
The result? A hybrid approach that's as robust as it is innovative. We're not just making predictions anymore; we're uncovering hidden patterns and decoding the secrets of the Montreal real estate market like never before. It's all about embracing the future while paying homage to the past, my friend.
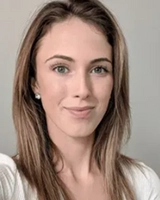
Integrate Real-Time Data Analysis
Integrating real-time data into traditional statistical techniques allows for a dynamic approach to analysis, making it possible to respond to trends and changes as they occur. This method enhances the responsiveness of a project by providing ongoing insights and updates, which is essential in fast-paced industries or scenarios where data evolves rapidly. By setting up systems to process and analyze data in real-time, project outcomes can reflect the most current information, leading to more accurate and timely decision-making.
Implementing such an approach requires careful planning to ensure data integrity and effective use of computational resources. Start leveraging real-time data to make your projects more adaptable and responsive.
Apply Robust Data Normalization
When dealing with data from a variety of sources, applying robust data normalization techniques is critical for ensuring that the information is comparable and usable. It is important to transform data into a common format, scale, or range, which then makes it possible to combine disparate data types and sources effectively. Data normalization helps in eliminating biases and anomalies that could lead to inaccurate conclusions.
This step in data preprocessing is particularly crucial when combining traditional statistics with data from modern, diverse datasets. Consider adopting appropriate data normalization methods to enhance the reliability of your statistical analyses.
Utilize Distributed Computing
Utilizing distributed computing can dramatically improve the scalability and efficiency of traditional statistical methods when applied to modern, large-scale projects. By spreading computational tasks across multiple machines or processors, larger datasets can be analyzed more rapidly than with a single processor approach. This distribution of work not only speeds up analysis but also enables the handling of complex computations that are typical in big data projects.
Distributed computing is a backbone of modern data analytics, and integrating it into statistical methodologies can provide the computational power necessary to derive insights from massive and intricate datasets. Explore distributed computing to take your data projects to the next level of scalability and performance.
Incorporate Bayesian Methods
Employing Bayesian methods in statistical analysis provides a framework for incorporating prior knowledge and handling uncertainty in a principled way. Bayesian techniques are adaptable to complex models and can update predictions or inferences as new data becomes available. This is especially beneficial in projects where the underlying systems or behaviors may not be fully understood, and uncertainty is a significant concern.
Such methods allow for a more nuanced understanding of data and better-informed decision-making. Investigate how Bayesian methods can be applied to your statistical analysis to better quantify and manage uncertainty in your projects.
Adopt Regularization Techniques
Regularization techniques are key tools to prevent overfitting in statistical models, ensuring that the inferences drawn are generalizable and not just tailored to a specific dataset. These techniques work by introducing a penalty for complexity, effectively keeping the models simpler and more robust. Regularization can be especially useful when dealing with modern datasets that may have many features or when the number of predictors is large relative to the number of observations.
By incorporating regularization, statistical models can maintain their predictive power without becoming overly complex or sensitive to small fluctuations in the data. Begin adopting regularization in your modeling process to safeguard against overfitting and improve the generalizability of your project outcomes.