What is the Process for Selecting the Most Appropriate Statistical Technique?
Statistician Zone

What is the Process for Selecting the Most Appropriate Statistical Technique?
Unlock the secrets of choosing the perfect statistical technique for your complex projects. In this article, experts share their insights on methods ranging from Multiple Regression Analysis to Mixed-Model Analysis. Spanning a total of seven practical tips, the journey begins with understanding how to select Multiple Regression Analysis and culminates in the wisdom of consulting with a statistician. Dive in to elevate your statistical decision-making process.
- Choose Multiple Regression Analysis
- Select Mixed-Model Analysis
- Define the Research Question
- Study Technique Requirements
- Categorize Data's Measurement Scale
- Focus on Robust and Efficient Methods
- Consult with a Statistician
Choose Multiple Regression Analysis
When working with a local SEO agency focused on improving Google Business Profiles, I faced a project where we needed to analyze various factors impacting our clients' rankings. The challenge was identifying which elements—like review frequency, image updates, or keyword usage—had the strongest influence on improving visibility. With multiple statistical methods available, the decision had to be precise.
To start, we gathered data across a range of clients, tracking their activities over a set period. We had several viable options, including regression analysis and correlation testing, to pinpoint patterns. After reviewing the data structure and variables involved, I chose multiple regression analysis. This technique allowed us to account for the influence of each variable while controlling for others, giving a clearer picture of what actions directly correlated with higher rankings.
By applying this method, we found that consistent photo uploads, combined with strategic keyword placements in business descriptions, had a more significant impact than initially thought. This insight helped us refine our approach, allowing clients to focus on activities proven to drive results, leading to higher satisfaction and improved retention.
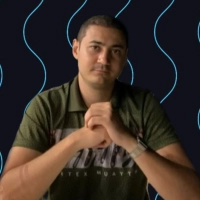
Select Mixed-Model Analysis
When faced with multiple viable statistical techniques for a project, my approach is always grounded in a thorough understanding of the data, the project's objectives, and the underlying assumptions of each method. With over 30 years of experience in musculoskeletal health and sports-injury rehabilitation, I've learned that selecting the right approach requires a combination of clinical insight and statistical knowledge. I start by evaluating the type of data we have—is it continuous, categorical, or a mix of both? Then, I consider the key outcomes we are aiming to achieve. For example, if we're examining injury patterns in athletes and want to understand the relationship between training loads and recovery times, I would lean towards regression analysis, especially if the data is continuous. However, if we're dealing with categorical outcomes like the presence or absence of a specific injury, logistic regression may be more appropriate.
One example that stands out is when I was working with elite dancers at The Alignment Studio. We were exploring the impact of different training routines on the frequency of lower-back pain. Initially, there were several potential methods to analyze the data, ranging from ANOVA to multiple regression. Given the complexity of the data, I chose a mixed-model analysis because it allowed us to account for both fixed effects, like training type, and random effects, like individual dancer variability. My academic background in physiotherapy and science, coupled with years of practical experience, gave me the confidence to select this approach. The result was a clear understanding of which training routines were contributing to lower-back pain, enabling us to adjust their programs and significantly reduce injury rates over time. This combination of clinical experience and methodological rigor was key to the project's success.
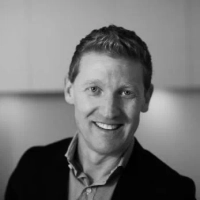
Define the Research Question
The process of choosing the right statistical technique starts with examining the research question. Understanding the question helps to outline the type of analysis required and shapes the direction of the entire study. It is essential to consider the nature of the collected data, such as whether it is categorical or continuous.
Identifying these characteristics early on aids in narrowing down potential methods. Considering the goals of the research will ensure alignment with the chosen technique. Take the first step by thoroughly defining the research question and type of data.
Study Technique Requirements
Evaluating the assumptions and limitations of various techniques is a crucial step in selecting the most fitting method. Different statistical methods come with their own sets of requirements, such as normality and homoscedasticity, which must be met for valid results. By understanding these conditions, researchers can avoid selecting methods that are incompatible with their data.
Some methods may also be more sensitive to outliers than others, influencing the choice. By closely examining these factors, better and more accurate results can be achieved. Begin by studying the requirements of potential techniques to match them with your data.
Categorize Data's Measurement Scale
Determining the measurement scale of the variables involved is essential for selecting an appropriate statistical technique. Whether the data covers nominal, ordinal, interval, or ratio scales directly impacts the range of suitable statistical methods. A mismatch between the data scale and the chosen technique could lead to incorrect conclusions.
Understanding the data's scale helps in choosing methods that can correctly analyze and interpret the information. Assessing the measurement scale early ensures the selection process is on solid ground. Start by categorizing your data's measurement scale accurately.
Focus on Robust and Efficient Methods
When considering statistical techniques, prioritizing those that offer robustness and efficiency can lead to more reliable results. Robust methods perform well even when certain assumptions are not fully met or when data contains outliers. Efficiency, on the other hand, involves selecting techniques that provide meaningful insights without excessive complexity or computation.
Balancing these factors ensures the analysis is both powerful and feasible. Emphasizing robustness and efficiency helps in achieving dependable outcomes. Focus on finding techniques that are both robust and efficient for your analysis needs.
Consult with a Statistician
Consulting with a statistician is often a valuable step in choosing the best statistical method. Statisticians bring expertise to the table, helping to clarify complex issues and provide tailored recommendations based on the specific needs of the research. They can address unique challenges and suggest the most appropriate techniques, ensuring a high-quality analysis.
This collaboration can save time and prevent common pitfalls encountered in statistical analysis. Seeking professional advice early in the process is highly beneficial. Reach out to a statistician to discuss your project's requirements in detail.